Publications
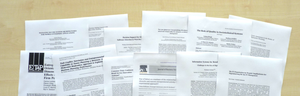
With our publications we cover the most diverse research areas that arise in the field of man, task and technology. In addition to traditional Business Information Systems topics such as knowledge management and business process management, you will also find articles on current topics such as blended learning, cloud computing or smart grids. Use this overview to get an impression of the range and possibilities of research in Business Information Systems at the University of Duisburg-Essen.
Type of Publication: Article in Journal
Does this Explanation Help? Designing Local Model-agnostic Explanation Representations and an Experimental Evaluation Using Eye-tracking Technology
- Author(s):
- Meza Martinez, Miguel Angel; Nadj, Mario; Langner, Moritz; Toreini, Peyman; Maedche, Alexander
- Title of Journal:
- ACM Transactions on Interactive Intelligent Systems
- Volume (Publication Date):
- 13 (2023)
- pages:
- 1-47
- Location(s):
- New York, NY, USA
- ISSN:
- 2160-6455
- Keywords:
- Machine learning, explainability, user-centric evaluation, eye-tracking
- Digital Object Identifier (DOI):
- doi:10.1145/3607145
- Citation:
- Download BibTeX
Abstract
In Explainable Artificial Intelligence (XAI) research, various local model-agnostic methods have been proposed to explain individual predictions to users in order to increase the transparency of the underlying Artificial Intelligence (AI) systems. However, the user perspective has received less attention in XAI research, leading to a (1) lack of involvement of users in the design process of local model-agnostic explanations representations and (2) a limited understanding of how users visually attend them. Against this backdrop, we refined representations of local explanations from four well-established model-agnostic XAI methods in an iterative design process with users. Moreover, we evaluated the refined explanation representations in a laboratory experiment using eye-tracking technology as well as self-reports and interviews. Our results show that users do not necessarily prefer simple explanations and that their individual characteristics, such as gender and previous experience with AI systems, strongly influence their preferences. In addition, users find that some explanations are only useful in certain scenarios making the selection of an appropriate explanation highly dependent on context. With our work, we contribute to ongoing research to improve transparency in AI.