Publications
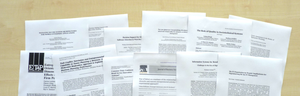
With our publications we cover the most diverse research areas that arise in the field of man, task and technology. In addition to traditional Business Information Systems topics such as knowledge management and business process management, you will also find articles on current topics such as blended learning, cloud computing or smart grids. Use this overview to get an impression of the range and possibilities of research in Business Information Systems at the University of Duisburg-Essen.
Type of Publication: Article in Collected Edition
Automatic online quantification and prioritization of data protection risks
- Author(s):
- Zmiewski, Sascha Sven; Laufer, Jan; Mann, Zoltán Ádám
- Title of Anthology:
- Proceedings of the 17th International Conference on Availability, Reliability and Security (ARES)
- Publisher:
- Association for Computing Machinery (ACM)
- Location(s):
- Vienna, Austria
- Publication Date:
- 2022
- ISBN:
- 9781450396707
- Keywords:
- vulnerability, self-adaptation, risk prioritization, risk assessment, data protection
- Digital Object Identifier (DOI):
- doi:10.1145/3538969.3539005
- Talk associated with this publication:
- 17th International Conference on Availability, Reliability and Security
- Talk associated with this publication:
- FoMSESS-Jahrestreffen
- Citation:
- Download BibTeX
Abstract
Data processing systems operate in increasingly dynamic environments, such as in cloud or edge computing. In such environments, changes at run time can result in the dynamic appearance of data protection vulnerabilities, i.e., configurations in which an attacker could gain unauthorized access to confidential data. An autonomous system can mitigate such vulnerabilities by means of automated self-adaptations. If there are several data protection vulnerabilities at the same time, the system has to decide which ones to address first. In other areas of cybersecurity, risk-based approaches have proven useful for prioritizing where to focus efforts for increasing security. Traditionally, risk assessment is a manual and time-consuming process. On the other hand, addressing run-time risks requires timely decision-making, which in turn necessitates automated risk assessment. In this paper, we propose a mathematical model for quantifying data protection risks at run time. This model accounts for the specific properties of data protection risks, such as the time it takes to exploit a data protection vulnerability and the damage caused by such exploitation. Using this risk quantification, our approach can make, in an automated process, sound decisions on prioritizing data protection vulnerabilities dynamically. Experimental results show that our risk prioritization method leads to a reduction of up to 15.8% in the damage caused by data protection vulnerabilities.